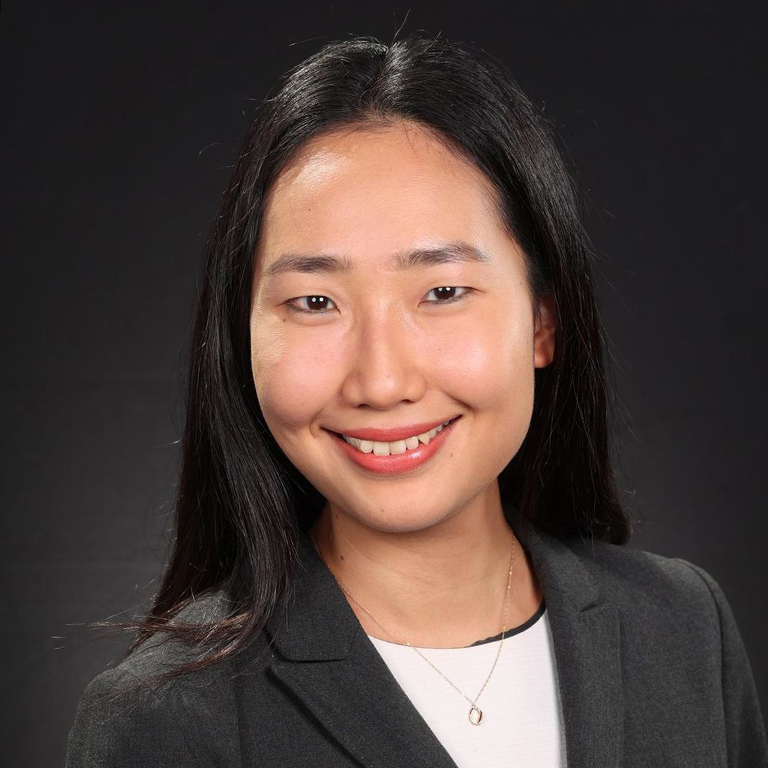
Jaymin Kim
Managing Director, Emerging Technologies, Global Cyber Insurance Center
-
United States
Since the 2022 launch of ChatGPT and other generative artificial intelligence (AI) technologies, the AI landscape has continued to develop, with new AI variations, including multimodal, agentic, and humanoid technologies.
Although often conflated with traditional AI, generative AI is just one subcategory of AI that primarily employs deep learning models to generate new content across various domains.
As organizations seek to manage the risks associated with generative AI, it is important to understand the ways that it can amplify existing risks, as well as the shifting legal, regulatory, and insurance implications related to its use. Some ideas around best practices and lessons have emerged in the past few years to help organizations navigate this formative phase.
AI refers to a diverse field of technologies that have existed since the 1950s. AI is broadly defined as computer systems that can simulate human intelligence, but there is variation in how these systems work and are used. For example:
Across these various subcategories of AI, there are some similarities but also critical differences in the kind of risk management and transfer solutions that may be used to mitigate corresponding risks. Another important consideration is who is using which kind of AI and for what purpose. For example, the risks can differ for an AI developer versus an end user.
This article will focus on generative AI specifically, as it is a relatively new form of AI that organizations are quickly adopting at scale. Agentic AI and humanoid AI are even newer forms of AI, for which the rate and scale of enterprise adoption remains to be seen.
Marsh’s extensive analyses to date suggest that the world has yet to observe a completely new category of risk that transpires from generative AI. Rather, generative AI may amplify existing risks, including cyberattacks, data privacy, intellectual property (IP), and the spread of misinformation.
Some of the early manifestations of generative AI risks include:
As the AI landscape develops, so too does the legal and regulatory environment, including emerging questions around IP, unfair competition, data privacy, and libel, in some cases with limited precedent. For example, one common issue is whether a generative AI model trained on copyrighted material constitutes infringement or unfair use. In the US, various court rulings have considered this question, with some dismissed, others upheld, and many pending. The US Copyright Office’s Copyright and Artificial Intelligence Report offers a helpful, early framework for understanding the emerging IP landscape in the US and beyond. It’s important to recognize that there are also relevant non-AI specific laws and regulations that continue to apply in the context of AI, including data privacy regulations.
Globally, some high-profile cases have brought the issue further into focus, such as France’s Autorité de la concurrence fining Google €250M for using news publishers’ content to train a generative AI model. Looking ahead, more cases like this one are expected to add to the legal conversation around copyright and IP.
Meanwhile, regulators worldwide are beginning to distinguish between AI and generative AI; a positive trend that acknowledges both the similarities and critical differences in corresponding risk mitigation. Mitigating traditional AI risks often includes a focus on model explainability and repeatability of outputs; this is technically challenging in the context of generative AI, for which risk mitigation shifts toward human oversight and iterative testing like red teaming.
There is also ongoing tension between the frameworks for addressing AI-related risks. More principles-based frameworks emphasize broad ethical guidelines and flexibility, while more rules-based frameworks, like the EU AI Act, establish specific, detailed regulations that must be followed, providing clearer compliance requirements. This distinction highlights the challenge of balancing regulatory oversight and technological advancement.
Leaders need to keep a close eye on changing laws and regulations to avoid unintentional missteps in an environment that is changing in real time.
For any organization using generative AI or developing generative AI models, key insurance considerations may include: How are we using it, and does our existing coverage potentially leave us exposed? For example, do we need to change limits and retentions on some lines, while leaving others untouched?
From a coverage perspective, many existing insurance products including casualty, media, cyber, and first-party insurance products, among others, offer cover for generative AI-related events. For example, a bodily injury event is still a bodily injury event regardless of whether a new technology was involved. That said, some limited policies and endorsements specific to generative AI are emerging, as well as more AI-specific underwriting questions, which suggest that having robust AI governance in place could become increasingly important, in the US and globally.
On the claims side, there have been few generative AI-specific claims to date. That said, some isolated incidents have gained attention, including one involving deepfake technology on a video call used to defraud a multinational firm of US$25 million.
Since January 2020, the proliferation of cyber exclusions in insurance has created significant protection gaps — known as silent cyber — by excluding some losses triggered by technological events. To avoid a similar situation with silent AI, organizations should recognize that generative AI can amplify risks and introduce new complexities within existing insurance lines.
It is important to confirm that technology triggers do not remove coverage intended by the policy, like removing IP coverage under an IP policy simply because AI or generative AI is a part of the causal link to loss. Evolving existing insurance contracts before creating new ones — and avoiding complexity in language — can help insureds and insurers align on coverage clarity and contract certainty.
To begin mitigating the risks associated with generative AI, organizations need to think comprehensively about how they develop, implement, and use this technology. The following three categories may offer a helpful starting point for implementing controls:
By implementing controls across these three categories, organizations may be able to more comprehensively mitigate and manage the risks associated with generative AI, with the goal of leading to more effective and sustainable use of the technology to achieve business outcomes.
There is considerable regulatory debate surrounding the use of copyrighted training data in generative AI. In theory, it is possible to develop generative AI models without using copyrighted data, or by obtaining the necessary licenses. If the use of copyrighted training data without appropriate licenses were to be deemed illegal, we could see various fines and penalties imposed on those who violate these regulations. However, it's important to note that this technology is here to stay, and these types of questions are ones we should be closely monitoring as laws and regulations may change over time.
There are numerous climate-related risks that accompany generative AI, as there are with many new technologies. Senior leaders should be providing appropriate oversight by actively discussing these issues and integrating them into their decision-making process. This could not only help mitigate potential management liability risks but also better align the organization with growing expectations for corporate responsibility in addressing climate concerns. Generative AI is here to stay, as are the environmental risks associated with it, so the sooner these discussions happen, the better.
As generative AI continues to present new opportunities for organizations, leaders must be attentive to its accompanying risks. Although there is still much to learn about generative AI, following are three steps organizations can take today to help strengthen their risk mitigation efforts.
Managing Director, Emerging Technologies, Global Cyber Insurance Center
United States
Global Cyber Product Leader and Head, Global Cyber Insurance Center
United States